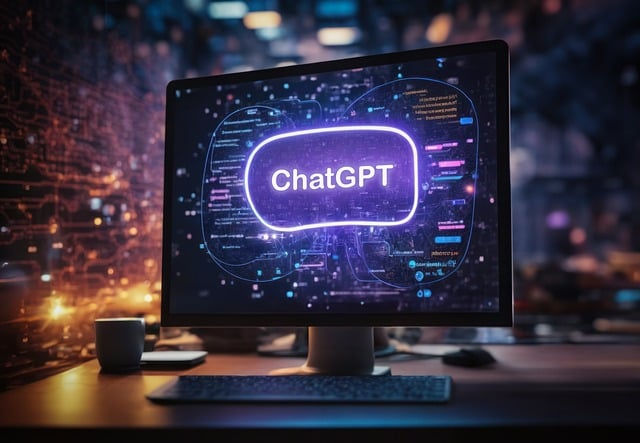
Since OpenAI released its artificial intelligence (AI) chatbot ChatGPT in October 2022, millions of Internet users have taken its help to write essays, thesis, poems, and codes. Its ability to pull relevant information from the web and summarize it for users in a simple language is seen by many as a precursor to a new era of chatbot-driven search.
Microsoft has followed suit, integrating GPT-4-powered chatbots and copilots across applications and Windows 11. Similarly, Google's Bard, now part of its search engine, offers similar functionalities.
These chatbots, alongside OpenAI's DALL-E and Codex models, share a common thread: multi-layer transformative neural networks, also known as transformers.
What is a transformer?
Developed in 2017 by researchers at Google and the University of Toronto, a transformer is a neural network used for natural language processing, natural language generation, and even genome sequencing.
A neural network is an interconnected group of artificial neurons based on the human brain. It is the underlying technology used by deep learning models to process complex data. Transformers are meant to process a piece of information and generate the most relevant response. What makes it more effective than earlier models is its ability to understand the link between two sequential data such as words in a sentence.
This allows it to understand the context even in long sentences and large paragraphs, something which was not possible with other models. For this, it uses mathematical techniques called attention or self-attention to identify how data elements influence or are linked to each other.
What was used before transformers?
Before transformers, most language models were built on something called a recurrent neural network (RNN), which processes data in a sequential manner. It is used for language translation, natural language processing (NLP), speech recognition, and image captioning. It is also used by Apple’s voice assistants Siri, Google Translate, and Google Duplex. The limitation of an RNN is that it processes every word in a sentence separately, which makes it difficult for it to handle long sentences or large paragraphs.
What is driving interest in transformers?
The release of chatGPT in October 2022 has triggered a scramble among organizations to tap into its underlying technology, known as the generative pre-trained transformer (GPT). Its third-generation model, GPT-3, was released in May 2020 and is said to be the largest language model ever trained. It is trained on 45TB of text data and has 175 billion parameters, which is ten times more than the 1.5 billion parameters of its predecessor GPT-2, which was trained on 40GB of text data.
Though GPT-3 uses the same architecture as its predecessor, it has more layers and it has been trained on a much larger dataset.
Data is the most critical element in any machine learning (ML) model, especially those based on complex neural networks. Larger datasets enable better classification and identification, which in turn makes the model better at performing its tasks.
GPT-3 is the reason why ChatGPT is so good at generating the most contextual and relevant information to any query.
After GPT-3 and GPT 3.5, OpenAI released a more advanced model called GPT-4, which can generate content from text and image prompts. OpenAI claims that the new model is more creative and collaborative, has better reasoning and capabilities and can handle much more nuanced instructions than GPT-3.5, which could only respond to text prompts. It is believed that GPT-4 has been trained on a much larger data set than GPT-3’s 175 billion data points, which is what makes it better at classification and identification. OpenAI is currently working on GPT-5 and is expected to launch it sometime this year.